Quantitative approaches to equity investing continue to introduce new sources of alpha. Recent advances in computing power, such as machine-learning and natural language processing are generating insights in areas formerly reserved for human research.
This has enabled increased analysis of non-traditional data sets that may provide valuable investment insights and a competitive edge amongst active equity investors.
Quantitative investing as we know it today originated in the 1980s with the formation of several quantitative investment firms, many of which are still thriving today. This fledgling industry benefited from a unique set of conditions:
- Financial data was now available in digital format.
- Computing power allowed them to do quantitative analysis that was previously incredibly tedious.
Fast forward to 2024, and portfolio optimizers now use sophisticated non-linear optimization algorithms, allowing them to handle complex functions and constraints more effectively. The algorithms have also become more efficient, using a technology called parallel processing that enables unprecedented scalability. This allows quant managers to optimize much larger portfolios with thousands of securities in their universes.
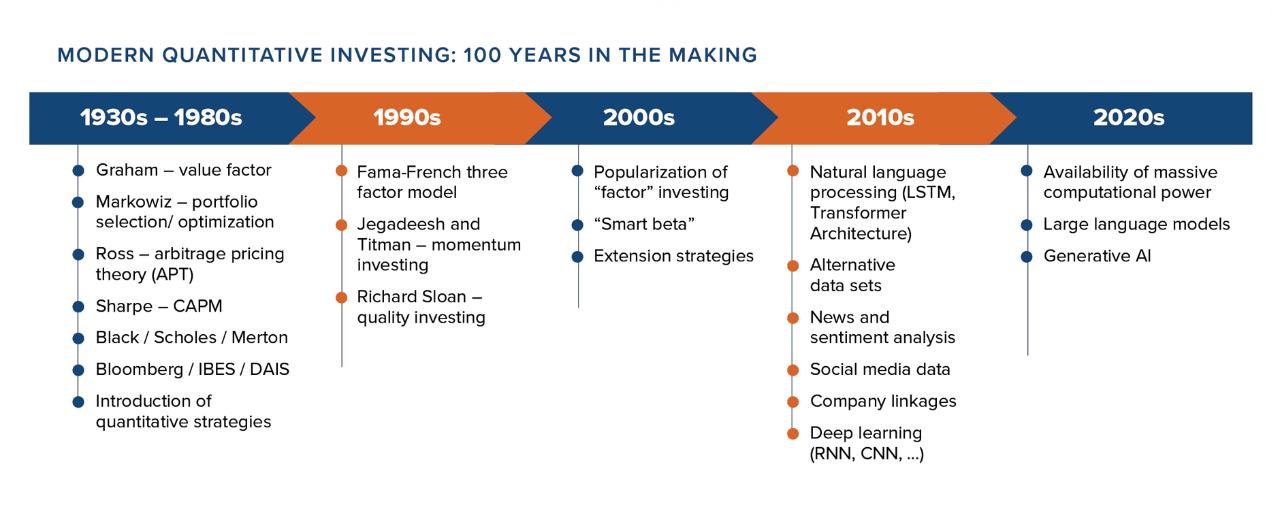
Today, we believe that most of the innovation in public equity investing is on the quantitative side. The convergence of computing power, novel data sets and new techniques allows portfolio managers to investigate and capture investment signals that were not previously available to them.
Many of these techniques are broadly categorized as “machine learning” (ML), a field of artificial intelligence that enables systems to identify patterns and make predictions from data. It also can learn from its experience without being explicitly programmed.
These technological advancements include:
Novel data sets: Non-traditional sources of data from which investors can generate investment insights.
Natural language processing (NLP): The analysis of text data using computers to extract information from text-based regulatory filings and earnings call transcripts.
Large language models (LLM): AI-powered language models that can be used to query information from a large set of textual data or analysis.
Generative AI: Models that can generate new, original content rather than simply analyzing existing data or making predictions.
Cloud computing/GPU computing: Allow quantitative investors to access massive computational power that allows quants to achieve in hours what used to take days.
These machine learning technologies all contribute toward the production of non-traditional investment signals. These signals produce scores for each stock in the given universe, which quants add to their investment models to predict future price movements.
Use of quantitative factors and alpha signals
Quantitative factors and investment signals are used to assess the attractiveness of each security.
One of the earliest known factors is value, identified by Benjamin Graham in “Security Analysis” (1934). Graham introduced the idea of using a number associated with a company (price-to-earnings ratio) to make investment decisions.
In 1992, Eugene Fama and Kenneth French introduced the Fama-French Three-Factor Model, which combined size, beta and value in a model to predict stock returns. The following year, Narasimhan Jegadeesh and Sheridan Titman laid the foundations for momentum investing in “Returns to Buying Winners and Selling Losers: Implications for Stock Market Efficiency”.
In the following years, academics and practitioners discovered more factors, falling into broad classifications of factors such as value, momentum, growth, quality and technical. Quants still rely heavily on academically supported factors as inputs to their models, but they increasingly use machine learning and novel-dataset-driven investment signals. The following table compares some common established factors with a few examples of newer investment signals.

Source: Mackenzie Global Quantitative Equity Team
The broad range of non-traditional investment signals discovered by quants is driving an increasing portion of the alpha within quantitative models and can also define a quant’s competitive edge. When researching new factors and investment signals, we believe good candidates for inclusion must have the following attributes:
- They need to make fundamental and intuitive sense.
- They need to have statistical significance in the predictive models they are part of.
- They should have persistent efficacy.
- They should have widespread efficacy across different geographies and sectors of the economy.
- They should be as uncorrelated to existing factors as possible.
The last point explains the continuous search for novel sources of data and new investment signals. With a proliferation of factors and signals, quantitative managers must carefully consider the implications of adding them to their models. A crude approach is to equally weight the predictions from multiple factors and then average them. Another simple but more effective approach is to use a linear regression model, which gives more weight to factors that have proven to be more predictive in the past.
Although perhaps counterintuitive, all these rapidly evolving tools, data sets and investment signals require more human oversight, not less. It is critical that quantitative investors apply their experience and expertise to the entire process to help ensure that their outputs make strong fundamental sense and lead to sound investment decisions.
As we continue to push the boundaries the pursuit of alpha will continue, perpetuating the relentless search for new sources of insight and opportunity.
Commissions, trailing commissions, management fees and expenses all may be associated with mutual fund investments. Please read the prospectus before investing. Mutual funds are not guaranteed, their values change frequently and past performance may not be repeated.
The content of this article (including facts, views, opinions, recommendations, descriptions of or references to, products or securities) is not to be used or construed as investment advice, as an offer to sell or the solicitation of an offer to buy, or an endorsement, recommendation or sponsorship of any entity or security cited. Although we endeavour to ensure its accuracy and completeness, we assume no responsibility for any reliance upon it.